We use Cloud SQL for our web applications. Previously, we used Microsoft Cloud, but we transitioned due to cost benefits. We find Google Cloud Platform (GCP) to be more cost-effective. For BigQuery, we store data in a message queue similar to Kafka, and when an event occurs, that data is triggered to be inserted into a BigQuery table through subscriptions.
Engineer at HTC Global Services (INDIA) Private
Efficient large dataset handling with seamless service integration
Pros and Cons
- "BigQuery allows for very fast access, and it is efficient in handling large datasets compared to other SQL databases."
- "There is a limitation when copying data directly from BigQuery; it only supports up to ten MB when copying data to the clipboard."
What is our primary use case?
How has it helped my organization?
We have seen significant improvements in data management processes, particularly with integration capabilities that allow us to easily retrieve and manipulate data through simple queries. This enhances our workflow significantly.
What is most valuable?
BigQuery allows for very fast access, and it is efficient in handling large datasets compared to other SQL databases. It integrates well with other GCP products, and creating subscriptions in the UI is straightforward. The whole ecosystem of GCP products makes BigQuery beneficial for our data-handling tasks. Additionally, it is more cost-effective compared to alternatives like AWS.
What needs improvement?
There is a limitation when copying data directly from BigQuery; it only supports up to ten MB when copying data to the clipboard. For larger data, we have to download it as JSON or Excel files. This limitation could be addressed for better usability.
Buyer's Guide
BigQuery
March 2025
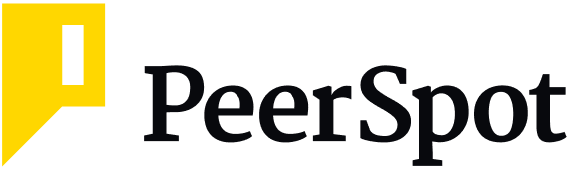
Learn what your peers think about BigQuery. Get advice and tips from experienced pros sharing their opinions. Updated: March 2025.
845,406 professionals have used our research since 2012.
For how long have I used the solution?
I have been working with Google Cloud SQL for over one year.
What do I think about the stability of the solution?
I have not experienced any downtime issues with the solution; it has been stable.
What do I think about the scalability of the solution?
There are some limitations in scalability, particularly when dealing with very large datasets. However, the cost savings we gain often balance this out.
How are customer service and support?
We haven't had much interaction with technical support outside of accessing documentation available online.
How would you rate customer service and support?
Positive
Which solution did I use previously and why did I switch?
Before using BigQuery, we were utilizing Azure Warehouse. We switched to BigQuery as GCP services were slightly cheaper and more cost-effective. The cost savings were a significant factor in our decision.
How was the initial setup?
The initial setup process for BigQuery was straightforward. By using Terraform, we were able to manage the entire setup efficiently and keep track of who is making changes.
What about the implementation team?
We have a team of developers who manage the platform. Infrastructure changes are tracked, and project owners approve updates.
What was our ROI?
I can't provide concrete documentation on ROI, but GCP's evolving services have been more cost-effective compared to AWS.
What's my experience with pricing, setup cost, and licensing?
AWS has a large number of users and has built a model with high costs, whereas GCP offers cost-effective solutions.
Which other solutions did I evaluate?
AWS was another option considered, but due to cost considerations, we opted for GCP.
What other advice do I have?
I recommend BigQuery, especially if you're already using GCP products, as the integration with other Google services is seamless.
I'd rate the solution nine out of ten.
Which deployment model are you using for this solution?
Public Cloud
If public cloud, private cloud, or hybrid cloud, which cloud provider do you use?
Google
Disclosure: I am a real user, and this review is based on my own experience and opinions.
Last updated: Nov 4, 2024
Flag as inappropriate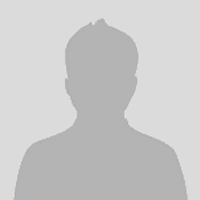
Lead Machine Learning Engineer at Schlumberger
A serverless system that is easy to set up and offers fast analysis of data
Pros and Cons
- "It's similar to a Hadoop cluster, except it's managed by Google."
- "It would be helpful if they could provide some dashboards where you can easily view charts and information."
What is our primary use case?
We are primarily using the solution to crunch data. Then, we are doing some ETL work on top of the data.
What is most valuable?
We like that it is a serverless system.
We can analyze terabytes of data in a very small amount of time.
It's similar to a Hadoop cluster, except it's managed by Google.
The initial setup is simple.
We find the product to be very stable.
It scales quite well.
What needs improvement?
If they can provide any charting platform on top of this product, that would be ideal. BigQuery now only allows us to run queries. It doesn't provide us with any insights. For example, if a query took so many times, they could maybe provide any suggestions on how to optimize the queries or speed up the process. It would be helpful if they could provide some dashboards where you can easily view charts and information. That would be very useful.
For how long have I used the solution?
I've been using the solution for two or three years.
What do I think about the stability of the solution?
This is a highly stable product. There are no bugs or glitches. It doesn't crash or freeze.
What do I think about the scalability of the solution?
The solution is very scalable.
Almost my entire team uses it. We have a 50-member team, and pretty much everyone is on it. They are mostly data engineers and developers.
How are customer service and support?
We have yet to reach out to technical support. We haven't had any issues.
Which solution did I use previously and why did I switch?
We chose this solution specifically since all of our services are in GCP, Google Cloud. Google Cloud has a basic internal coupling with BigQuery. That's the reason we are using BigQuery.
How was the initial setup?
The initial setup is very easy. You just have to log in to the Google Cloud console, and then you can just create a few tables and start using it.
From start to finish it takes about half an hour. It is even less than that to get the tables up and running. The deployment is quite fast.
What's my experience with pricing, setup cost, and licensing?
I'm not sure about the exact cost, however, it is charged on the queries which you run, basically. For example, if you run a query, the amount of data scanned through BigQuery will dictate the costs.
What other advice do I have?
I am a customer and end-user.
I'm not sure which version of the solution we're using.
It's a serverless platform deployed on a public cloud.
I'd advise potential users to set up their tables accordingly. There are two sets of optimization that BigQuery provides as well. You set up whichever columns you want to do the partition and on which columns you want to do the clustering. If these columns are defined properly, then BigQuery's a breeze to use.
On a scale from one to ten, I would rate it at an eight. If they just added a few more features, it would be almost perfect.
Which deployment model are you using for this solution?
Public Cloud
If public cloud, private cloud, or hybrid cloud, which cloud provider do you use?
Google
Disclosure: I am a real user, and this review is based on my own experience and opinions.
Buyer's Guide
BigQuery
March 2025
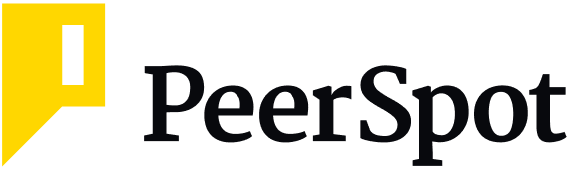
Learn what your peers think about BigQuery. Get advice and tips from experienced pros sharing their opinions. Updated: March 2025.
845,406 professionals have used our research since 2012.
Red Team Operator at Argentina Red Team
Analyzes logs from systems to identify the severity of issues but lacks integrations
Pros and Cons
- "BigQuery excels at data analysis. It processes vast amounts of information using its advanced architecture and sophisticated querying capabilities, making it crucial for critical insights and safe for handling sensitive data."
- "BigQuery should integrate with other tools, such as Cloud Logging and Local Studio, to enhance its capabilities further and enable powerful and innovative analyses."
What is our primary use case?
BigQuery allows you to quickly analyze logs from your systems to identify the severity of issues. It integrates well with other Google Cloud services, such as Cloud Logging, where you can easily manipulate various data types and analyze all logs.
What is most valuable?
BigQuery excels at data analysis. It processes vast amounts of information using its advanced architecture and sophisticated querying capabilities, making it crucial for critical insights and safe for handling sensitive data.
What needs improvement?
BigQuery should integrate with other tools, such as Cloud Logging and Local Studio, to enhance its capabilities further and enable powerful and innovative analyses.
For how long have I used the solution?
I have been using BigQuery for two years.
Which solution did I use previously and why did I switch?
I have opted for Fireye, Elasticsearch, and Alcon. One principal difference is that BigQuery starts with machine learning and WAN implementations, while you can implement VMware or other active boxes. Therefore, it is recommended that cloud VMs be used for BigQuery processes. You can execute jobs in the cloud, such as VMware.
For instance, you can compute analytics for email, apply filters, and manipulate weather data. It provides higher efficiency, though exact benchmarks are unclear. Additionally, starting the query flow login request can also be advantageous.
How was the initial setup?
The initial setup is automatic. It requires one person. You need to log in to the Google Cloud platform, import the necessary package into your query, and then you can start querying your data.
If you need a solid CRM solution integrated with Azure, you'll need knowledgeable people to support it. Three individuals can form a strong CRM team connected to Azure, leveraging BigQuery.
What was our ROI?
You can use BigQuery to generate and manage large datasets efficiently. Whether using a flexible integrated environment like Dataflow or a local studio, BigQuery provides powerful tools for querying and analyzing data.
What's my experience with pricing, setup cost, and licensing?
The product is free of cost.
What other advice do I have?
Setting up BigQuery on GCP is crucial. When creating a service account, you define the permissions required for project identification or access monitoring systems.
You configure policies using IAM roles to manage access permissions effectively within GCP. These roles govern the service accounts created for specific tasks such as data processing, system monitoring, or other service integrations. When you activate these policies, a JSON token is generated. This token can authenticate and authorize access to Google services like BigQuery or other third-party applications.
Moreover, by configuring VMs to match data processing requirements, you ensure that the data is securely handled by the applications associated with the service accounts. This setup enables seamless communication between your applications and Google services, facilitating efficient data acquisition and processing.
Overall, I rate the solution a seven out of ten.
Which deployment model are you using for this solution?
Public Cloud
Disclosure: I am a real user, and this review is based on my own experience and opinions.
Data Quality Specialist at a energy/utilities company with 201-500 employees
Facilitate data exploration with centralized data and table visualization
Pros and Cons
- "Its integration with other tools like Atlan through a Google Chrome extension is highly beneficial."
- "It can be slower and more problematic compared to other platforms such as Snowflake."
What is our primary use case?
I usually need to catalog. In my case, it's more related to data governance. I need to catalog information from BigQuery. I want to ensure the data quality tool is in sync with BigQuery, so I go to BigQuery and do queries to make sure it was synced with Atlan, for example, for data quality tools. I create validation rules and need to write the rule in BigQuery to create a query there, see how long it takes to run, and evaluate its performance in a data quality tool.
How has it helped my organization?
What I have seen is that they are using BigQuery as a central repository. They bring dispersed information to BigQuery, which facilitates exploring the data and gaining insights. Consequently, it improves operations, response time, and the business overall.
What is most valuable?
As a user, I have liked using BigQuery to create queries. They have a table explorer feature that allows you to select a table, choose fields, and generate queries easily, which significantly facilitates my workflow. I also appreciate the lineage feature, which shows how tables relate to each other and enables end-to-end usage visualization.
Furthermore, its integration with other tools like Atlan through a Google Chrome extension is highly beneficial. Using BigQuery's central repository brings dispersed information together, which facilitates exploring the data and gaining insights. Consequently, it improves operations, response time, and the business overall.
What needs improvement?
There are integration challenges, particularly with performance when exporting data to BigQuery from other tools like Qualitics. It can be slower and more problematic compared to other platforms such as Snowflake.
For how long have I used the solution?
I have been working with BigQuery for one year.
What do I think about the stability of the solution?
I have not seen a lot of problems, so I would say BigQuery is quite stable.
What do I think about the scalability of the solution?
In my opinion, BigQuery is very scalable yet has some limitations regarding performance that are not always as required.
How are customer service and support?
I don't have direct contact with BigQuery's support team. Our organization manages this through internal communication, and I contact my company’s team when issues arise.
How would you rate customer service and support?
Positive
What other advice do I have?
I would recommend using BigQuery because it's a very good tool, easy to manage, and similar to other databases. Those familiar with SQL Server or Oracle can adapt to BigQuery easily. It's a scalable cloud solution.
Overall, I would rate BigQuery as nine out of ten.
Disclosure: I am a real user, and this review is based on my own experience and opinions.
Last updated: Dec 3, 2024
Flag as inappropriateAssociate Consultant (Data Engineer) at MediaAgility
Provides flexibility and is competitively priced
Pros and Cons
- "The most valuable features of BigQuery is that it supports standard SQL and provides good performance."
What is our primary use case?
We use BigQuery to perform data warehouse migration for clients willing to move to GCP from their on-premise solution.
What is most valuable?
The solution's pricing is really competitive compared to other peers. The most valuable features of BigQuery is that it supports standard SQL and provides good performance.
For how long have I used the solution?
I have been using BigQuery for three years.
What do I think about the stability of the solution?
I rate BigQuery a nine out of ten for stability.
What do I think about the scalability of the solution?
Around 30 to 40 users use BigQuery in our organization.
I rate BigQuery ten out of ten for scalability.
Which solution did I use previously and why did I switch?
I previously worked with Microsoft SQL Server.
How was the initial setup?
The solution’s initial setup is very easy. You just have to spin up a data set and start using it.
I rate BigQuery ten out of ten for the ease of its initial setup.
What about the implementation team?
The solution can be deployed by one person in a few minutes.
What's my experience with pricing, setup cost, and licensing?
The solution's pricing is cheaper compared to other solutions. On a scale from one to ten, where one is cheap, and ten is expensive, I rate the solution's pricing a two or three out of ten.
What other advice do I have?
Potential users can trust BigQuery without any second thoughts. The solution's pricing is great compared to other solutions. The solution provides more flexibility and supports standard SQL, and anyone coming out from a different platform would not face any challenges adopting BigQuery.
Overall, I rate BigQuery a nine out of ten.
Disclosure: I am a real user, and this review is based on my own experience and opinions.
Sr Manager at a transportation company with 10,001+ employees
Everything they advertised worked exactly as promised
Pros and Cons
- "We basically used it to store server data and generate reports for enterprise architects. It was a valuable tool for our enterprise design architect."
- "I would like to see version-based implementation and a fallback arrangement for data stored in BigQuery storage. These are some features I'm interested in."
What is our primary use case?
We basically used it to store server data and generate reports for enterprise architects. It was a valuable tool for our enterprise design architect.
What is most valuable?
Everything they advertised or listed worked exactly as promised. That was advantageous to us.
What needs improvement?
In future releases, I would like to see more pre-defined aggregated forms. After using BigQuery, we need to use the data in an enterprise architecture dimensional data model. So, having pre-defined aggregated forms would be helpful.
Additionally, I would like to see version-based implementation and a fallback arrangement for data stored in BigQuery storage. These are some features I'm interested in.
For how long have I used the solution?
I have experience with BigQuery.
What about the implementation team?
When I joined the company, BigQuery was already implemented by our team.
What's my experience with pricing, setup cost, and licensing?
It is a cheap solution.
What other advice do I have?
I would recommend getting a clear understanding of BigQuery's functionalities and what it's best suited for. If your needs align with its capabilities, then you should definitely proceed.
BigQuery offers fantastic features, but it's important to understand its purpose beforehand. Otherwise, you might face difficulties later on.
Overall, I would rate the solution an eight out of ten.
Which deployment model are you using for this solution?
Public Cloud
If public cloud, private cloud, or hybrid cloud, which cloud provider do you use?
Google
Disclosure: I am a real user, and this review is based on my own experience and opinions.
Full-stack Developer at ViewersLogic
Fast, flexible, scalable, stable, and easy to learn
Pros and Cons
- "What I like most about BigQuery is that it's fast and flexible. Another advantage of BigQuery is that it's easy to learn."
- "An area for improvement in BigQuery is its UI because it's not working very well. Pricing for the solution is also very high."
What is our primary use case?
My company uses BigQuery as a data warehouse.
What is most valuable?
What I like most about BigQuery is that it's fast and flexible.
Another advantage of BigQuery is that it's easy to learn.
You can also use it from anywhere.
What needs improvement?
An area for improvement in BigQuery is its UI because it's not working very well.
Pricing for the solution is also very high.
In general, though, I like the solution very much.
For how long have I used the solution?
I've been using BigQuery for six months now.
What do I think about the stability of the solution?
I found BigQuery stable in my six months of using it, and I'd rate its stability as ten out of ten.
What do I think about the scalability of the solution?
BigQuery is a scalable solution, and it's a nine out of ten in terms of scalability.
How are customer service and support?
I've never interacted with BigQuery support.
Which solution did I use previously and why did I switch?
We used Redshift as a database for our operations, but now, we've moved to BigQuery because BigQuery is much more than a database. It has more features than Redshift, and we hope to pay less than what we paid when we were using Redshift because Redshift required us to pay ahead each month, and the total cost was too much.
How was the initial setup?
BigQuery was easy to set up, but you'll need to learn how to do it. In general, the initial setup is straightforward.
I'd rate the BigQuery setup as eight out of ten.
What about the implementation team?
Our in-house team implemented BigQuery for the company.
What's my experience with pricing, setup cost, and licensing?
BigQuery pricing can increase quickly. It's a high-priced solution.
It would help if you researched how to reduce the price. It would take some time to find out how to set up BigQuery in a way that reduces its pricing.
What other advice do I have?
My company is using a data warehouse solution called BigQuery.
My advice to anyone deciding on using BigQuery is to be aware of the pricing mechanism and have a better understanding of it to avoid surprises. You pay for what you use, so it could be very easy to lose control, which means the BigQuery costs could go up fast.
I'd rate BigQuery as nine out of ten.
Disclosure: I am a real user, and this review is based on my own experience and opinions.
Data Engineering and AI Intern at .3Lines Venture Capital
Good solution for large databases that require a lot of analytics
Pros and Cons
- "BigQuery is a powerful tool for managing and analyzing large datasets. The versatility of BigQuery extends to its compatibility with external data visualization tools like Power BI and Tableau. This means you not only get query results but can also seamlessly integrate and visualize your data for better insights."
- "Some of the queries are complex and difficult to understand."
What is our primary use case?
BigQuery is a powerful tool for managing and analyzing large datasets. The versatility of BigQuery extends to its compatibility with external data visualization tools like Power BI and Tableau. This means you not only get query results but can also seamlessly integrate and visualize your data for better insights.
What is most valuable?
The product's most valuable feature is its ability to connect to visualization tools.
What needs improvement?
Some of the queries are complex and difficult to understand.
For how long have I used the solution?
I have been using the product for more than a year.
What do I think about the scalability of the solution?
My company has 100 users for BigQuery.
How are customer service and support?
The tool's support is fast to respond.
How would you rate customer service and support?
Positive
How was the initial setup?
The tool's deployment is easy if you follow Google's documentation.
What other advice do I have?
If you have a big database and lots of analytics, BigQuery is a really good tool. It helps save and manage your queries and gives you results you can show clients and others. I rate it a nine out of ten.
Disclosure: I am a real user, and this review is based on my own experience and opinions.
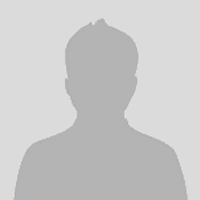
Buyer's Guide
Download our free BigQuery Report and get advice and tips from experienced pros
sharing their opinions.
Updated: March 2025
Product Categories
Cloud Data WarehousePopular Comparisons
Azure Data Factory
Teradata
Snowflake
Microsoft Azure Synapse Analytics
Vertica
Dremio
Oracle Autonomous Data Warehouse
AWS Lake Formation
SAP Business Warehouse
Yellowbrick Cloud Data Warehouse
Buyer's Guide
Download our free BigQuery Report and get advice and tips from experienced pros
sharing their opinions.
Quick Links
Learn More: Questions:
- Which ETL or Data Integration tool goes the best with Amazon Redshift?
- What are the main differences between Data Lake and Data Warehouse?
- What are the benefits of having separate layers or a dedicated schema for each layer in ETL?
- What are the key reasons for choosing Snowflake as a data lake over other data lake solutions?
- Are there any general guidelines to allocate table space quota to different layers in ETL?
- What cloud data warehouse solution do you recommend?
- Can you please help me understand cloud databases?
- When evaluating Cloud Data Warehouse, what aspect do you think is the most important to look for?
- bitmap index as preferred choice in data warehousing environment
- Why do you recommend using a cloud data warehouse?