I'm a customer. I'm using Azure Data Factory.
DGM - Business Intelligence at a comms service provider with 1,001-5,000 employees
Cloud integration and flexible data handling meet our needs effectively
Pros and Cons
- "I find that the solution integrates well with cloud technologies, which we are using for different clouds like Snowflake and AWS"
- "I do not have any notes for improvement."
What is our primary use case?
What is most valuable?
I find that the solution integrates well with cloud technologies, which we are using for different clouds like Snowflake and AWS. It is much more flexible in terms of transferring data from on-premise or on cloud. There is no need to create different mappings for different tables. The platform has the capability to handle metadata efficiently. So all our needs are being fulfilled with the platform we have right now.
What needs improvement?
I do not have any notes for improvement.
For how long have I used the solution?
I have used it for almost six years.
Buyer's Guide
Azure Data Factory
June 2025
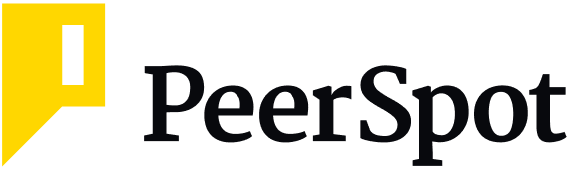
Learn what your peers think about Azure Data Factory. Get advice and tips from experienced pros sharing their opinions. Updated: June 2025.
860,592 professionals have used our research since 2012.
What do I think about the stability of the solution?
The stability is quite good.
Which solution did I use previously and why did I switch?
We wanted to move on to the cloud.
How was the initial setup?
The initial setup is almost not difficult for technical people.
What other advice do I have?
I used to work on Informative Support Center. Now we are using Azure Data Factory.
I rate it eight out of ten.
Which deployment model are you using for this solution?
Public Cloud
If public cloud, private cloud, or hybrid cloud, which cloud provider do you use?
Disclosure: My company does not have a business relationship with this vendor other than being a customer.
Last updated: Jan 17, 2025
Flag as inappropriate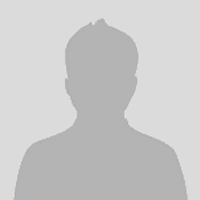
CTO at a construction company with 1,001-5,000 employees
The data factory agent is quite good but pricing needs to be more transparent
Pros and Cons
- "The data factory agent is quite good and programming or defining the value of jobs, processes, and activities is easy."
- "The pricing model should be more transparent and available online."
What is our primary use case?
Our company uses the solution as a data pipeline. We get information outside the cloud from our factory such as data relating to production. We categorize it, clean it up, and transfer it to a database and data model. From there, we analyze the data using BI and other things. We gather information in data lake products like Microsoft Synapse and Microsoft Data Lake.
We have two to three administrators who use the solution in a quite standard, mainstream way with nothing extreme. They handle administration, security, and development.
It is difficult to define the total number of users because that depends on the number of data factory agents. We built the solution to have a different data factory agent for every customer. For example, if we have ten customers then we have ten users. We hope to increase usage but growth depends on our marketing efforts and how well we sell our products.
What is most valuable?
The data factory agent is quite good and programming or defining the value of jobs, processes, and activities is easy. We have the agent installed on-premises in order to gather information.
The cloud includes all kinds of API connections so we can easily gather information from other services.
The solution seamlessly integrates with the Azure infrastructure.
What needs improvement?
The pricing model should be more transparent and available online. When you start programming, you define the fields, variables, activities, and components but don't know the implication on price. You get a general idea but the more activities you add, the more you pay. It would be better to know price implications up front.
There is a calculator you can run to simulate price but it doesn't help a lot. Practically speaking, you have to build your job and run it to see the exact price implications. This is an issue because you might realize you are paying too much so you have to reprogram or change things.
For how long have I used the solution?
I have been using the solution for three years.
What do I think about the stability of the solution?
The solution is stable with no issues. Stability is rated a nine out of ten.
We did have some breaches, but that was because we misconfigured something. Since we corrected it, we haven't had any issues.
What do I think about the scalability of the solution?
The solution is scalable with no performance issues. We haven't yet reached our limit that would require scaling. Scalability is rated an eight out of ten.
How are customer service and support?
We have discussions with our Microsoft partner all the time.
In the last three years, we have contacted Microsoft directly three or four times. Once was for a general architectural issue and the rest were for the data factory or other items. Each time, we talked together with Microsoft and our partner.
Support gave us answers and solved our issues. Sometimes, we didn't like the answer but we accepted that it was the correct answer.
Support is rated a nine out of ten.
How would you rate customer service and support?
Positive
Which solution did I use previously and why did I switch?
We have not used another solution to this magnitude for real development and production. We work a little bit on Google Cloud.
How was the initial setup?
The initial setup was quite quick. Deployment was fairly simple and took less than a week. The setup got us up and running.
After that, we had to write the implications of the data model and the kinds of activities. We are still doing this today because we make changes all the time.
What about the implementation team?
The initial setup was seamless because we worked with a gold star Microsoft partner. Our side of setup was pretty quiet. We talked with our partner and told them what we needed from a security and monitoring point of view. We had a few high-level discussions from the block diagram perspective. Basically we said we need this or that, and our partner made it happen.
The team included one person from our partner and three in-house team members with varying expertise across data modeling, security, and devops. We always worked with the same person but maybe behind the scenes he talked with coworkers. He did talk several times with Microsoft but we don't really know how many people were involved.
The solution does not require infrastructure maintenance. If we ever have issues, we can use Azure Defender to resolve them. We only make slight changes at the application level.
What was our ROI?
We haven't calculated ROI on a formal level, but the fact is we need the solution. Because of the integration, we save a lot but haven't run exact numbers.
What's my experience with pricing, setup cost, and licensing?
The pricing model is based on usage and is not cheap. Based on our activity, we pay about $2,000 per month.
Pricing is rated a four out of ten.
Which other solutions did I evaluate?
If we didn't have the solution, we would have to find another tool because data pipelines are an essential part of our business.
The biggest advantage to the solution is its integration with the Azure infrastructure that includes the active directory, security, Synapse, Data Lake, Power BI, and the data factory agent.
All of the integration was a big consideration for us. We had general guidelines that said working with one vendor would provide the best integrations. The guideline was to use Microsoft unless there was an issue.
We did not look at a third party or open source even though there are similar tools available.
What other advice do I have?
My best advice is to keep an eye on the pricing because we found out the hard way. Pricing is actually related to the way you use what the solution calls activity. This activity stuff drastically changes the coding to the rate you gather information from your client environment.
So, when marketing guys tell you to gather information every minute, you have to weigh the heavy implications in comparison to collecting data once an hour or day. Programmers and developers designed the solution based on usage activity and building tasks or jobs.
Pay a lot of attention to the pricing implications from the starting point of view. Technically, you can solve all issues but you need to keep an eye on the pricing.
From a technical point of view, the solution is rated an eight out of ten. Because of pricing, the solution's overall rating is downgraded to a seven out of ten.
Which deployment model are you using for this solution?
Public Cloud
If public cloud, private cloud, or hybrid cloud, which cloud provider do you use?
Microsoft Azure
Disclosure: My company does not have a business relationship with this vendor other than being a customer.
Buyer's Guide
Azure Data Factory
June 2025
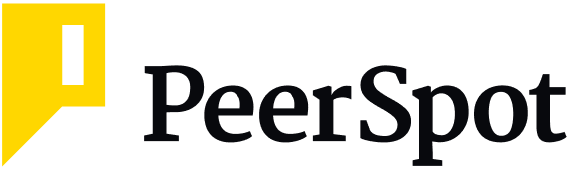
Learn what your peers think about Azure Data Factory. Get advice and tips from experienced pros sharing their opinions. Updated: June 2025.
860,592 professionals have used our research since 2012.
Data Architect at World Vision
The good, the bad and the lots of ugly
Pros and Cons
- "The trigger scheduling options are decently robust."
- "There is no built-in pipeline exit activity when encountering an error."
What is our primary use case?
The current use is for extracting data from Google Analytics into Azure SQL Database as a source for our EDW. Extracting from GA was problematic with SSIS.
The larger use case is to assess the viability of the tool for larger use in our organization as a replacement for SSIS for our EDW and also as an orchestration agent to replace SQL Agent for firing SSIS packages using Azure SSIS-IR.
The initial rollout was to solve the immediate problem while assessing its ability to be used for other purposes within the organization. And also establish the development and administration pipeline process.
How has it helped my organization?
ADF allowed us to extract Google Analytics data (via BigQuery) without purchasing an adapter.
It has also helped with establishing how our team can operate within Azure using both PaaS and IaaS resources and how those can interact. Rolling out a small data factory has forced us to understand more about all of Azure and how ADF needs to rely upon and interact with other Azure resources.
It provides a learning ground for use of DevOps Git along with managing ARM templates as well as driving the need to establish best practices for CI.
What is most valuable?
The most valuable aspect has been a large list of no-cost source and target adapters.
It is also providing a PaaS ELT solution that integrates with other Azure resources.
Its graphical UI is very good and is even now improving significantly with the latest preview feature of displaying inner activities within other activities such as forEach and If conditions.
Its built-in monitoring and ability to see each activity's JSON inputs/outputs provide an excellent audit trail.
The trigger scheduling options are decently robust.
The fact that it's continually evolving is hopeful that even if some feature is missing today, it may be soon resolved. For example, it lacked support for simple SQL activity until earlier this year, when that was resolved. They have now added a "debug until" option for all activities. The Copy Activity Upsert option did not perform well at all when I first started using the tool but now seems to have acceptable performance.
The tool is designed to be metadata driven for large numbers of patterned ETL processes, similar to what BIML is commonly used for in SSIS but much simpler to use than BIML. BIML now supports generating ADF code although with ADF's capabilities I'm not sure BIML still holds its same value as it did for SSIS.
What needs improvement?
The list of issues and gaps in this tool is extensive, although as time goes on, it gets shorter. It currently includes:
1) Missing email/SMTP activity
2) Mapping data flows requires significant lag time to spin up spark clusters
3) Performance compared to SSIS. Expect copy activity to take ten times that of what SSIS takes for simple data flow between tables in the same database
4) It is missing the debug of a single activity. The workaround is setting a breakpoint on the task and doing a "rerun from activity" or setting debug on activity and running up to that point
5) OAuth 2.0 adapters lack automated support for refresh tokens
6) Copy activity errors provide no guidance as to which column is causing a failure
7) There's no built-in pipeline exit activity when encountering an error
8) Auto Resolve Integration runtime should never pick a region that you're not using (should be your default for your tenant)
9) IR (integration runtime) queue time lag. For example, a small table copy activity I just ran took 95 seconds of queuing and 12 seconds to actually copy the data. Often the queuing time greatly exceeds the actual runtime
10) Activity dependencies are always AND (OR not supported). This is a significant missing capability that forces unnecessary complex workarounds just to handle OR situations when they could just enhance the dependency to support OR like SSIS does. Did I just ask when ADF will be as good as SSIS?
They need to fix bugs. For example:
1) The debug sometimes stops picking up saved changes for a period of time, rendering this essential tool useless during that time
2) Enable interactive authoring (a critical tool for development) often doesn't turn on when enabled without going into another part of the tool to enable it. Then, you have to wait several minutes before it's enabled which is time you're blocked from development until it's ready. And then it only activates for up to 120 minutes before you have to go through this all over again. I think Microsoft is trying to torture developers
3) Exiting the inside of an activity that contains other activities always causes the screen to jump to the beginning of a pipeline requiring re-navigating where you were at (greatly slowing development productivity)
4) Auto Resolve Integration runtime (using default settings) often picks remote regions (not necessarily even paired regions!) to operate, which causes either an unnecessary slowdown or an error message saying it's unable to transfer the volume of data across regions
5) Copy activity often gets the error "mapping source is empty" for no apparent reason. If you play with the activity such as importing new metadata then it's happy again. This sort of thing makes you want to just change careers. Or tools.
For how long have I used the solution?
I have been using this product for six months.
What do I think about the stability of the solution?
Production operation seems to run reliably so far, however, the development environment seems very buggy where something works one day and not the next.
What do I think about the scalability of the solution?
So far, the performance of this solution is abysmal compared to SSIS. Especially with small tasks such as copying activity from one table to another within the same database.
How are customer service and support?
Customer support is non-existent. I logged multiple issues only to hear back from 1st level support weeks later asking questions and providing no help other than wasting my time. In one situation it was a bug where the debug function stopped working for a couple of days. By the time they got back to me, the problem went away.
How would you rate customer service and support?
Negative
Which solution did I use previously and why did I switch?
We have been and still rely on SSIS for our ETL. ADF seems to do ELT well but I would not consider it for use in ETL at this time. Its mapping data flows are too slow (which is a large understatement) to be of practical use to us. Also, the ARM template situation is impractical for hundreds of pipelines like we would have if we converted all our SSIS packages into pipelines as a single ADF couldn't take on all our pipelines.
How was the initial setup?
Initial setup is the largest caveat for this tool. Once you've organized your Azure environment and set up DevOps pipelines, the rest is a breeze. But this is NOT a trivial step if you're the first one to establish the use of ADF at your organization or within your subscription(s). Instead of learning just an ETL tool, you have to get familiar with and establish best practices for the entire Azure and DevOps technologies. That's a lot to take on just to get some data movements operational.
What about the implementation team?
I did this in-house with the assistance of another team who uses DevOps with Azure for other purposes (non-ADF use).
What's my experience with pricing, setup cost, and licensing?
The setup cost is only the time it takes to organize Azure resources so you can operate effectively and figure out how to manage different environments (dev/test/sit/UAT/prod, etc.). Also, how to enable multiple developers to work on a single data factory without losing changes or conflicting with other changes.
Which other solutions did I evaluate?
We operate only with SSIS today, and it works very well for us. However, looking toward the future, we will need to eventually find a PaaS solution that will have longer sustainability.
Which deployment model are you using for this solution?
Public Cloud
If public cloud, private cloud, or hybrid cloud, which cloud provider do you use?
Microsoft Azure
Disclosure: My company does not have a business relationship with this vendor other than being a customer.
Technical Manager at PalTech
Provides orchestration and data flows for transformation for integration
Pros and Cons
- "The data flows were beneficial, allowing us to perform multiple transformations."
- "When we initiated the cluster, it took some time to start the process."
What is our primary use case?
We use the solution for building a few warehouses using Microsoft services.
How has it helped my organization?
We worked on a project for the textile industry where we needed to build a data warehouse from scratch. We provided a solution using Azure Data Factory to pull data from multiple files containing certification information, such as CSV and JSON. This data was then stored in a SQL Server-based data warehouse. We built around 30 pipelines in Azure Data Factory, one for each table, to load the data into the warehouse. The Power BI team then used this data for their analysis.
What is most valuable?
For the integration task, we used Azure Data Factory for orchestration and data flows for transformation. The data flows were beneficial, allowing us to perform multiple transformations. Additionally, we utilized web API activities to log data from third-party API tools, which greatly assisted in loading the necessary data into our warehouse.
What needs improvement?
When we initiated the cluster, it took some time to start the process. Most of our time was spent ensuring the cluster was adequately set up. We transitioned from using the auto integration runtime to a custom integration runtime, which showed some improvement.
For how long have I used the solution?
I have been using Azure Data Factory for four years.
What do I think about the stability of the solution?
When running the process server, we encountered frequent connection disconnect issues. These issues often stemmed from internal problems that we couldn’t resolve then, leading to repeated disruptions.
I rate the stability as seven out of ten.
What do I think about the scalability of the solution?
20 people are using this solution daily. I rate the scalability around eight out of ten.
How are customer service and support?
Customer service supported us whenever we needed it.
How would you rate customer service and support?
Positive
Which solution did I use previously and why did I switch?
We have used SQL Server.
How was the initial setup?
The initial setup is easy and takes four to five hours to complete.
What was our ROI?
They have reduced the infrastructure burden by 60 percent.
What's my experience with pricing, setup cost, and licensing?
Pricing is reasonable when compared with other cloud providers.
What other advice do I have?
We have used the Key value pair for authentication with the adoption. I can rate it around eight out of ten.
I recommend the solution.
Overall, I rate the solution a nine out of ten.
Disclosure: My company does not have a business relationship with this vendor other than being a customer.
Director - Emerging Technologies at Speridian Technologies
Helps to orchestrate workflows and supports both ETL and ELT processes
Pros and Cons
- "Data Factory allows you to pull data from multiple systems, transform it according to your business needs, and load it into a data warehouse or data lake."
- "While it has a range of connectors for various systems, such as ERP systems, the support for these connectors can be lacking."
What is our primary use case?
Azure Data Factory is primarily used to orchestrate workflows and move data between various sources. It supports both ETL and ELT processes. For instance, if you have an ERP system and want to make the data available for reporting in a data lake or data warehouse, you can use Data Factory to extract data from the ERP system as well as from other sources, like CRM systems.
Data Factory allows you to pull data from multiple systems, transform it according to your business needs, and load it into a data warehouse or data lake. It also supports complex data transformations and aggregations, enabling you to generate summary and aggregate reports from the combined data. Data Factory helps you ingest data from diverse sources, perform necessary transformations, and prepare it for reporting and analysis.
How has it helped my organization?
I have extensive experience building things independently, with over twenty years of experience in SQL, ETL, and data-related projects. Recently, I have been using Azure Data Factory for the past two years. It has proven to be quite effective in handling large volumes of data and performing complex calculations. It allows for the creation of intricate data workflows and processes faster. Azure Data Factory is particularly useful for enterprise-level data integration activities, where you might deal with millions of records, such as in SAP environments. For example, SAP tables can contain tens or hundreds of millions of records. Managing and maintaining the quality of this data can be challenging, but Azure Data Factory simplifies these tasks significantly.
What is most valuable?
It is a powerful tool and is considered one of the leading solutions in the market, especially for handling large volumes of data. It is popular among large enterprises.
What needs improvement?
While it has a range of connectors for various systems, such as ERP systems, the support for these connectors can be lacking. Take the SAP connector, for example. When issues arise, it can be challenging to determine whether the problem is on Microsoft's side or SAP's side. This often requires working with both teams individually, which can lead to coordination issues and delays. It would be beneficial if Azure Data Factory provided better support and troubleshooting resources for these connectors, ensuring a smoother resolution of such issues.
For how long have I used the solution?
I have been using Azure Data Factory for two years.
What do I think about the stability of the solution?
I rate the solution's stability a nine out of ten.
What do I think about the scalability of the solution?
It's pretty good. There are no issues with scalability.
How are customer service and support?
The support has been good.
How would you rate customer service and support?
Positive
How was the initial setup?
It is straightforward to set up. However, ensuring its security requires careful configuration, which can vary depending on the organization's requirements. While the basic setup is user-friendly and doesn’t necessarily require advanced technical skills, securing the environment involves additional steps to prevent unauthorized access and ensure that data is only accessible from permitted locations. This can be more complex depending on the specific setup and organizational needs.
Setting up the infrastructure typically takes about two to three weeks and usually requires the effort of two people.
What was our ROI?
Azure Data Factory serves several important purposes. One key reason for using it is to build an enterprise data warehouse. This is crucial for centralizing data from various sources. Another reason is to gain insights from that data. By consolidating data in a unified location, you enable data scientists and engineers to analyze it and generate valuable insights.
Customers use Azure Data Factory to bring their data together, creating opportunities to understand their data better and extract actionable insights. However, simply consolidating data is not enough; the actual value comes from how you analyze and utilize it. This involves deriving insights, creating opportunities, and understanding customers better, which can significantly benefit the organization.
What's my experience with pricing, setup cost, and licensing?
Pricing is fine. It's a pay-as-you-go option.
It is in the same price range as other major providers. However, costs can vary depending on enterprise agreements and relationships.
What other advice do I have?
Overall, I rate the solution a nine out of ten.
Disclosure: My company has a business relationship with this vendor other than being a customer. Partner
Solution Architect at Giant Eagle
Easy to use and can be used for data integration
Pros and Cons
- "The most valuable features of the solution are its ease of use and the readily available adapters for connecting with various sources."
- "Some known bugs and issues with Azure Data Factory could be rectified."
What is our primary use case?
We use Azure Data Factory for data integration.
What is most valuable?
The most valuable features of the solution are its ease of use and the readily available adapters for connecting with various sources.
What needs improvement?
Some known bugs and issues with Azure Data Factory could be rectified.
For how long have I used the solution?
I have been using Azure Data Factory for about two years.
What do I think about the stability of the solution?
I rate the solution an eight out of ten for stability.
What do I think about the scalability of the solution?
Azure Data Factory is a scalable solution. A team of 16 people from the data analytics team use the solution in our organization.
I rate the solution an eight out of ten for scalability.
How was the initial setup?
On a scale from one to ten, where one is difficult and ten is easy, I rate the solution's initial setup a seven out of ten.
What about the implementation team?
A team of three people deployed Azure Data Factory in three to four days.
What's my experience with pricing, setup cost, and licensing?
The solution's pricing is competitive.
What other advice do I have?
We build data pipelines primarily for integration. Few of them are real-time data transfers, and few of them would be a batch-free file. These would direct the data from various sources to our data warehouse. Azure Data Factory helps build the data pipelines and adaptors.
The solution has built-in features and a control center for us to monitor the status of the pipelines. The solution's email notification also helps us in monitoring. We didn't face any challenges to set up the data pipelines. We know there are some controls, but governance is customized for the organization's requirements. We have our own policies.
Azure Data Factory is deployed on the cloud in our organization. I would recommend Azure Data Factory to other users.
Overall, I rate the solution a nine out of ten.
Disclosure: My company does not have a business relationship with this vendor other than being a customer.
Company Owner, Data Consultant at Telenet BVBA
An expensive data tool for migration with Data Catalog
What is our primary use case?
We use the solution for migration. We collect data from SAP and various other sources, including multiple ERP systems. These ERP systems encompassed different versions of SAP, Dynamics, Navision, and Oracle, presenting a considerable challenge for data integration. The objective was to consolidate all data into Azure Data Factory and Data Warehouse, establishing a structured framework for reporting and analytics. The main hurdle encountered was data ingestion, particularly with SAP data, due to its significant volume. Alternative tools such as PolyBase were utilized to expedite the process, as standard SAP APIs were insufficient for loading data into Azure Data Services. Collaboration with an Azure data engineer facilitated the exploration of alternative ingestion methods.
What is most valuable?
The most important feature is the Data Catalog. We need to define all the data fields we test. It has technical information in the Data Catalog. The main feature is data ingestion in ADF. We also extended it to PurView because PurView is an extension of the Azure data catalog. It can scan metadata. There is a limitation in ADF when setting up the data catalog.
What needs improvement?
Integration with other tools, such as SAP, could be enhanced. It still has challenges when we talk about different types of structured and non-structured datages. Azure Data Factory has data ingestion issues. There are no delays out of the box. We needed a lot of tools to make the ingestion happen because of the data structure and size of the data.
The transformation we needed to do on data was also not so easy. It was also a long process. We had a bit more capabilities for setting up the Data Catalog, but it still didn't solve the problem from the data ingestion.
For how long have I used the solution?
I have been using Azure Data Factory as a consultant for five years.
What do I think about the stability of the solution?
Sometimes, we experienced some instability, mainly on injection.
I rate the solution’s stability as seven out of ten.
What do I think about the scalability of the solution?
I rate the solution’s scalability an eight out of ten.
How are customer service and support?
The support is very good.
How would you rate customer service and support?
Positive
How was the initial setup?
You need to be experienced in deploying the solution. It's not so easy for a business user. Depending on the use case, it takes around six months to get a proof of concept done.
I rate the initial setup a seven out of ten, where one is easy and ten is difficult.
What's my experience with pricing, setup cost, and licensing?
The pricing is visible because you pay for what you do.
The product looks quite expensive because it charges based on the size of the data. If you're not aware, your cost can be very high. If you are experienced, you know that.
I rate the product’s pricing a seven out of ten, where one is cheap and ten is expensive.
What other advice do I have?
I was mainly focusing on ingestion and cataloging. Data engineers were handling data orchestration.
The tool’s maintenance is easy.
There could be a bit more clarity in the pricing structure. It should be understandable for business users. The cost is is becoming too high because users are unaware of the pricing structure. Secondly, the tool should integrate better with other tools like ERP systems.
Overall, I rate the solution a seven out of ten.
Disclosure: My company has a business relationship with this vendor other than being a customer. MSP
Associate Specialist at Synechron
We can integrate our Databricks notebooks and schedule them
Pros and Cons
- "ADF is another ETL tool similar to Informatica that can transform data or copy it from on-prem to the cloud or vice versa. Once we have the data, we can apply various transformations to it and schedule our pipeline according to our business needs. ADF integrates with Databricks. We can call our Databricks notebooks and schedule them via ADF."
- "I rate Azure Data Factory six out of 10 for stability. ADF is stable now, but we had problems recently with indexing on an SQL database. It's slow when dealing with a huge volume of data. It depends on whether the database is configured as general purpose or hyperscale."
What is our primary use case?
We are currently migrating from on-prem to the cloud, and our on-prem tables are getting data from upstream. We used ADF to build a pipeline to facilitate this migration. A team of 15-20 people currently uses ADF, and more will join once it goes live.
What is most valuable?
ADF is another ETL tool similar to Informatica that can transform data or copy it from on-prem to the cloud or vice versa. Once we have the data, we can apply various transformations to it and schedule our pipeline according to our business needs. ADF integrates with Databricks. We can call our Databricks notebooks and schedule them via ADF.
For how long have I used the solution?
I have used Azure Data Factory for about six months.
What do I think about the stability of the solution?
I rate Azure Data Factory six out of 10 for stability. ADF is stable now, but we had problems recently with indexing on an SQL database. It's slow when dealing with a huge volume of data. It depends on whether the database is configured as general purpose or hyperscale.
How was the initial setup?
I rate Azure Data Factory eight out of 10 for ease of setup. The deployment time depends on the data volume. Four million records will take longer than four thousand. Migrating our full load from on-prem to the cloud took around 16-18 hours because the volume was 17 million.
What's my experience with pricing, setup cost, and licensing?
I rate ADF six out of 10 for affordability. The cost depends on the services we use. It's usage-based.
What other advice do I have?
I rate Azure Data Factory seven out of 10. Companies that want to migrate from on-prem to the cloud have lots of options. I haven't explored them all, but Azure, GCP, and AWS are essentially all the same.
Which deployment model are you using for this solution?
Public Cloud
If public cloud, private cloud, or hybrid cloud, which cloud provider do you use?
Microsoft Azure
Disclosure: My company does not have a business relationship with this vendor other than being a customer.
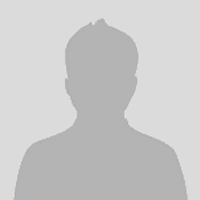
Buyer's Guide
Download our free Azure Data Factory Report and get advice and tips from experienced pros
sharing their opinions.
Updated: June 2025
Popular Comparisons
Informatica Intelligent Data Management Cloud (IDMC)
Informatica PowerCenter
Teradata
Snowflake
Oracle Data Integrator (ODI)
Palantir Foundry
IBM InfoSphere DataStage
Talend Open Studio
Oracle GoldenGate
SAP Data Services
Qlik Replicate
OpenText Analytics Database (Vertica)
Buyer's Guide
Download our free Azure Data Factory Report and get advice and tips from experienced pros
sharing their opinions.
Quick Links
Learn More: Questions:
- Which solution do you prefer: KNIME, Azure Synapse Analytics, or Azure Data Factory?
- How do Alteryx, Denod, and Azure Data Factory overlap (or complement) each other?
- Do you think Azure Data Factory’s price is fair?
- What kind of organizations use Azure Data Factory?
- Is Azure Data Factory a secure solution?
- How does Azure Data Factory compare with Informatica PowerCenter?
- How does Azure Data Factory compare with Informatica Cloud Data Integration?
- Which is better for Snowflake integration, Matillion ETL or Azure Data Factory (ADF) when hosted on Azure?
- What is the best suitable replacement for ODI on Azure?
- Which product do you prefer: Teradata Vantage or Azure Data Factory?