I use the solution in my company for some product prediction purposes and in the retail processes. My company collects data in Azure and Cynet. My company develops some ML algorithms in Microsoft Azure Machine Learning Studio. Two years ago, I had a training session with Microsoft, so now I can develop ML algorithms in the retail sector.
Senior Expert Data Science Product Owner at Boyner Buyuk Magazacilik A.S.
Helps users develop ML algorithms easily
Pros and Cons
- "The product's initial setup phase is easy."
- "The high price of the product is an area of concern where improvements are required."
What is our primary use case?
What needs improvement?
The high price of the product is an area of concern where improvements are required. The product needs to be available at a cheaper price. I think the tool needs to be made available to children for free, especially if you have a student's or a teacher's email ID from a university.
For how long have I used the solution?
I have been using Microsoft Azure Machine Learning Studio for two years. I am a user of the solution.
What do I think about the stability of the solution?
It is a stable solution. I have seen a lot of products, but I feel that Microsoft Azure Machine Learning Studio is the most stable tool in the market. Stability-wise, I rate the solution an eight out of ten.
Buyer's Guide
Microsoft Azure Machine Learning Studio
March 2025
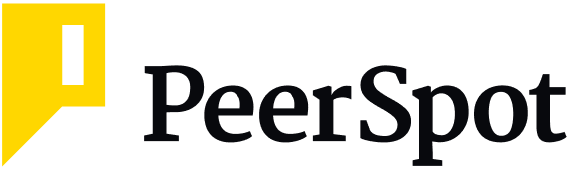
Learn what your peers think about Microsoft Azure Machine Learning Studio. Get advice and tips from experienced pros sharing their opinions. Updated: March 2025.
845,406 professionals have used our research since 2012.
What do I think about the scalability of the solution?
It is a scalable solution. Scalability-wise, I rate the solution an eight out of ten.
For development purposes, there are ten people, and since my institute expanded its use, I think it will increase to fifty.
I plan to increase the tool's usage.
How are customer service and support?
I got support for the tool in Turkey with the help of Mircorsoft's admins and consultants and they were okay. I rate the technical support an eight out of ten.
How would you rate customer service and support?
Positive
Which solution did I use previously and why did I switch?
I evaluated DataIQ against Microsoft Azure Machine Learning Studio. Most of the applications I used were small or niche tools, while Microsoft Azure Machine Learning is huge and expandable.
How was the initial setup?
The product's initial setup phase is easy. I rate the product's initial setup phase as an eight out of ten.
The solution is deployed using Azure's cloud services.
The solution time for deployment can take a few hours. I work in an enterprise with a medium range of data.
What's my experience with pricing, setup cost, and licensing?
I rate the product price as a nine on a scale of one to ten, where ten means it is very expensive.
What other advice do I have?
You can take care of the input of the datasets, and then Microsoft Azure Machine Learning Studio will provide everything for you. The tool has drag-and-drop options, and it becomes easy to develop anything, acting as a time-saver.
The drag-and-drop options in Microsoft Azure Machine Learning Studio influence the machine learning workflow as it allows you to put in some links, and it integrates with nearly all of your systems. You can use the datasets with the tool program to see the features and dimensions of all the datasets. Then you can choose some ML algorithm about the datasets, and it recommends the program, after which you can test a lot of models, but normally, you can't do it because it's not in Python or something else, leaving you confused. In Microsoft Azure Machine Learning Studio, there is a system from beginning to end that covers everything one should do.
The tool does have an influence on performance as it makes things faster and more productive.
I can recommend the tool to my colleagues. It provides the developer or product owner the ability to watch the system overall. If you use the tool you can begin learning about ML and you become more effective and faster which is important for enterprise.
I rate the tool a nine out of ten.
If public cloud, private cloud, or hybrid cloud, which cloud provider do you use?
Microsoft Azure
Disclosure: I am a real user, and this review is based on my own experience and opinions.
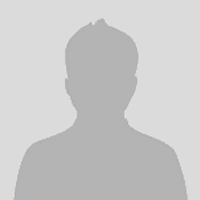
Technical Director at Integral Solutions (Asia) Pte Ltd
A solution to help deal with cross-selling and upselling activities that need to include generative AI in its future release
Pros and Cons
- "The most valuable feature of the solution is the availability of ChatGPT in the solution."
- "Stability-wise, you may face certain problems when you fail to refresh the data in the solution."
What is our primary use case?
My company uses Microsoft Azure Machine Learning Studio to help our company's customers view AI solutions.
My company's clients' use cases will be that they use the solution to feed information to the system about their customers who purchase from them. The solution also helps one to combine products to engage in cross-selling and upselling activities while keeping track of customer lifetime value. The solution also helps its users with the pricing simulation part to figure out what prices are good for the business and maximize the closing of the sale.
What is most valuable?
The most valuable feature of the solution is the availability of ChatGPT in the solution.
What needs improvement?
Improvement will be possible with more machine learning functionalities in Microsoft Azure Machine Learning Studio since, at times, the current accuracy of the solution is not good enough. It would be good if Microsoft Azure Machine Learning Studio could have a generative AI tool similar to ChatGPT.
For how long have I used the solution?
I have been using Microsoft Azure Machine Learning Studio for three years. My company functions as a reseller and a partner of Microsoft.
What do I think about the stability of the solution?
Stability-wise, I rate the solution a seven out of ten. With ML, you may face some data-related issues, especially considering that when dealing with customers at times, the data that comes in might not be clean. Stability-wise, you may face certain problems when you fail to refresh the data in the solution.
What do I think about the scalability of the solution?
Scalability-wise, I rate the solution a seven out of ten. My company still has to do some of our own optimizations to the data part of the solution until and unless we subscribe to some third-party data lake services, which is a better option but comes at a higher cost.
My company's client's organization has around 10 to 50 users of the solution.
My company caters to the requirements of medium and enterprise-sized companies.
How are customer service and support?
I rate the technical support a six out of ten.
How would you rate customer service and support?
Neutral
How was the initial setup?
I rate the initial setup phase of the solution a six on a scale of one to ten, where one is difficult, and ten is easy. The initial setup phase of the solution was a bit complex. The setup phase is a bit difficult if you want to view Microsoft Azure Machine Learning Studio as an application.
The solution is deployed 50 percent on the cloud and 50 percent on-premises.
Considering the fact that my company currently builds some standard solutions, Microsoft Azure Machine Learning Studio's deployment takes us around two to three months.
What's my experience with pricing, setup cost, and licensing?
I rate the solution's pricing a four on a scale of one to ten, where one is cheap, and ten is expensive.
There are some additional payments to be made apart from the licensing fees of the solution since buying Microsoft Azure Machine Learning Studio alone won't make it a complete solution. You will need the database and data lake services.
What other advice do I have?
Microsoft Azure Machine Learning Studio does not allow users to have a PnP option, like an ERP or a CRM system, where everything works if you include the data with the system. Sometimes, it is difficult to generate good patterns using the solution. You need to have good experience with the solution to move around with the data from the beginning before coming up with different strategies to end different problems. In general, the product is not a straightforward solution.
There is a need for Microsoft Azure Machine Learning Studio's users to put in some programming efforts to make the solution work accurately under different scenarios.
I rate the overall solution a six out of ten.
Which deployment model are you using for this solution?
Hybrid Cloud
Disclosure: My company has a business relationship with this vendor other than being a customer: Reseller
Buyer's Guide
Microsoft Azure Machine Learning Studio
March 2025
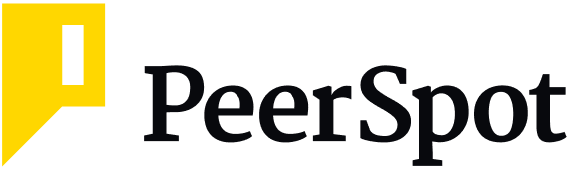
Learn what your peers think about Microsoft Azure Machine Learning Studio. Get advice and tips from experienced pros sharing their opinions. Updated: March 2025.
845,406 professionals have used our research since 2012.
Data Scientist at Sunergy
Empowers developers to build, deploy, and manage high-quality models faster
Pros and Cons
- "In the Machine Learning Studio, particularly the Designer part, which is essentially Azure's demo designer, there is room for improvement. Many customers and users tend to switch to Microsoft Azure Multi-Joiners, which is a more basic version, but they do so internally. One area that could use enhancement is the process of connecting components. Currently, every time you want to connect a component, such as linking it to your storage or an instance like EC2, you have to input your username and password repeatedly. This can be quite cumbersome. Google, for instance, has made it more user-friendly by allowing easy access for connecting services within a workspace. In a workspace, you can set up various resources like storage, a database cluster, machine learning studio, and more. When connecting these services, there's no need to enter your username and password each time, making it a more efficient process. Another aspect to consider is the role of the designer, and they were to integrate a large language model to handle various tasks, it could significantly enhance the overall scalability and usability of the platform."
What is our primary use case?
I've had experience working for two distinct companies. My previous employer operated in the telecom domain, primarily focused on telecom-related projects. In my current role, which is in the shipping domain, we primarily manage shipping cases. Furthermore, our current work predominantly revolves around a machine learning platform implemented on storage systems.
How has it helped my organization?
Performing tasks in a cloud service is incredibly straightforward. It offers excellent scalability and provides both JUPITAN and designer environments. There's no need to write extensive code; instead, you can simply drag and drop elements and connect components effortlessly. This allows for the creation of end-to-end workflows with minimal effort. It's a user-friendly and scalable solution, which is why I prefer working with it. Additionally, it allows for effective version control management.
What needs improvement?
In the Machine Learning Studio, particularly the Designer part, which is essentially Azure's demo designer, there is room for improvement. Many customers and users tend to switch to Microsoft Azure Multi-Joiners, which is a more basic version, but they do so internally.
One area that could use enhancement is the process of connecting components. Currently, every time you want to connect a component, such as linking it to your storage or an instance like EC2, you have to input your username and password repeatedly. This can be quite cumbersome. Google, for instance, has made it more user-friendly by allowing easy access for connecting services within a workspace. In a workspace, you can set up various resources like storage, a database cluster, machine learning studio, and more. When connecting these services, there's no need to enter your username and password each time, making it a more efficient process. Another aspect to consider is the role of the designer, and they were to integrate a large language model to handle various tasks, it could significantly enhance the overall scalability and usability of the platform.
For how long have I used the solution?
I have been working with Microsoft Azure Machine Learning Studio for three years.
What do I think about the stability of the solution?
I would rate it eight out of ten.
What do I think about the scalability of the solution?
I would rate it eight out of ten.
Which solution did I use previously and why did I switch?
Yes, I have worked with Azure in my previous experiences.
How was the initial setup?
The initial setup duration largely depends on your prior experience with the service. While setting up is generally straightforward, the time-consuming part comes in when you have to repeatedly input your username and password to connect with different building blocks. The deployment time can vary significantly. If you opt for an internal deployment suggested by Azure, it's relatively quick. However, if you're looking for an external deployment, it might take more time. The deployment timeline hinges on the project's scope and architecture.
Based on my experience, I find that it typically doesn't require a substantial amount of time.
In my previous experience using Azure and Machine Learning Studio, the database service offers an integrated option for data cleaning and ETL. This means you don't need to allocate extra time for data preparation and deployment because everything is interconnected. Monitoring progress is also feasible. Therefore, in terms of deployment and data engineering, there's generally not a significant increase in time required unless the project scope is extensive. For moderately scaled projects, a single person can handle the entire deployment.
The initial setup is moderate and I would rate it seven out of ten.
What's my experience with pricing, setup cost, and licensing?
There isn’t any such expensive costs and only a standard license is required.
What other advice do I have?
It is a good solution and will prove to be very helpful for your project. I would recommend it and rate it seven out of ten.
Which deployment model are you using for this solution?
Private Cloud
Disclosure: I am a real user, and this review is based on my own experience and opinions.
Practice Director at Birlasoft IndiaLtd.
Enables quick development of solutions, particularly those that are text analytics and cognitive-based
Pros and Cons
- "Auto email and studio are great features."
- "Using the solution requires some specific learning which can take some time."
What is our primary use case?
The use cases of this product are primarily for the BFSI; digitization and building machine learning models that provide recommendations for creating analytical insights from extracted data. We also do Jupyter Notebook authoring. We are partners with Microsoft and I'm a practice director.
How has it helped my organization?
The product enables quick data preparation and data processing pipeline as well as modeling work and it's all part of Azure Machine Learning. It also gives us an idea of what machine learning model is good to use because the hyperparameter tuning is done automatically which saves us time and effort.
What is most valuable?
Auto email and the studio are great features.
What needs improvement?
It's not that easy to master the program, it requires some specific learning. If we want to extend the program to include inexperienced users, it can take some time for them to learn the solution. It would be nice if they added GPU solutions. Most of the solutions coming out now are video analytics or edge computing-based and Azure should have that focus.
What do I think about the stability of the solution?
We haven't had any issues with stability.
What do I think about the scalability of the solution?
We haven't faced any challenges with scalability. If there are any issues, our Microsoft infract team pitches in but we haven't had any serious problems. We have around 25 to 30 customers accessing this solution. Maintenance is straightforward and doesn't require more than one person.
How are customer service and support?
Customer support is very good, they are prompt and helpful in solving problems.
How would you rate customer service and support?
Positive
Which solution did I use previously and why did I switch?
Our switch to AMLS was an organic development that came from the needs of our customers and was based on the quick time to develop and the pre-built machine learning models that the solution has.
How was the initial setup?
The initial setup is straightforward with deployment time depending on the environment. It depends on how many machine learning models we need to develop, the type of resources, the different sources, data volumes, etc.
What's my experience with pricing, setup cost, and licensing?
We don't deal with licensing, that is something our customers are responsible for. My understanding is that the cost is $50 for the digitization of 1,000 pages. I think it should be reduced to somewhere between $20 to $30 per 1,000 pages so that we can make a better offer to our customers.
What other advice do I have?
I believe Azure Machine Learning has a very good pre-built model which enables quick development of solutions, particularly text analytics and cognitive-based solutions.
I rate this solution nine out of 10.
Which deployment model are you using for this solution?
Public Cloud
Disclosure: My company has a business relationship with this vendor other than being a customer: Partner
Solution Sales Specialist at Intent Solutions Group
Seamless integration boosts workflow efficiency while pricing needs reevaluation
Pros and Cons
- "The integration with Azure services enhances workflow and meets my expectations."
- "The platform as a service provides user-friendly instruments, making the experience easy."
- "The pricing policy should be improved."
- "The pricing policy should be improved. I find the pricing to be not a good story in this case, as it is not affordable for everyone."
What is our primary use case?
I use Microsoft Azure Machine Learning Studio as a component in more complex stories involving Azure AI and Azure integration with business needs.
What is most valuable?
I can smoothly integrate with other Azure services, which is a valuable feature. The platform as a service provides user-friendly instruments, making the experience easy.
Additionally, the integration with Azure services enhances workflow and meets my expectations.
What needs improvement?
The pricing policy should be improved. I find the pricing to be not a good story in this case, as it is not affordable for everyone.
For how long have I used the solution?
I have been using Microsoft Azure Machine Learning Studio for about two years.
What do I think about the stability of the solution?
Stability is high, with a rating of nine out of ten.
What do I think about the scalability of the solution?
Microsoft Azure Machine Learning Studio is scalable.
How are customer service and support?
Microsoft technical support is rated a seven out of ten. There used to be better times for Microsoft technical support.
How would you rate customer service and support?
Neutral
How was the initial setup?
The initial setup involves provisioning and configuration rather than installation. It is a short story, taking about ten minutes per service for proper configuration.
What about the implementation team?
My team of specialists handles the implementation. We are a system integrator with a team of around two hundred engineers, twenty-five of whom work in AI and data.
What was our ROI?
Measuring ROI is hard due to the nature of projects, however, Microsoft provides a business case builder to help estimate potential profits and values from its products.
What's my experience with pricing, setup cost, and licensing?
Pricing is considered to be top-segment and should be improved. I rate the pricing as three or four on a scale of one to ten in terms of affordability.
What other advice do I have?
I would recommend trying and using it as it will be okay. I rate the overall solution eight out of ten.
Which deployment model are you using for this solution?
Public Cloud
If public cloud, private cloud, or hybrid cloud, which cloud provider do you use?
Microsoft Azure
Disclosure: My company has a business relationship with this vendor other than being a customer:
Last updated: Dec 29, 2024
Flag as inappropriateLead Technical Instructor at Code.Hub
A well organized solution that helps to create pipelines in minutes
Pros and Cons
- "The product is well organized. The thing is how we will get the models to work within our code. We have some suggestions there, but we want to gain more experience and be ready to answer that because we are currently working on this and don't have all the answers yet. The tool is well organized. What I am very happy about is the ease of deploying new resources. You can easily create your pipeline within minutes."
- "One problem I experience is that switching between multiple accounts can be difficult. I don't think there are any major issues. Mostly, the biggest challenge is to identify business solutions to this. The tool should keep on updating new algorithms and not stay static."
What is our primary use case?
We have data from our business, and we want to make AI models. The question is how we want to use those models in our business. That's what we're going to do next year.
What is most valuable?
The product is well organized. The thing is how we will get the models to work within our code. We have some suggestions there, but we want to gain more experience and be ready to answer that because we are currently working on this and don't have all the answers yet.
The tool is well organized. What I am very happy about is the ease of deploying new resources. You can easily create your pipeline within minutes.
What needs improvement?
One problem I experience is that switching between multiple accounts can be difficult. I don't think there are any major issues. Mostly, the biggest challenge is to identify business solutions to this.
The tool should keep on updating new algorithms and not stay static.
For how long have I used the solution?
I have been working with the product for ten years.
What do I think about the stability of the solution?
I rate Microsoft Azure Machine Learning Studio's stability as nine out of ten.
What do I think about the scalability of the solution?
I rate the solution's scalability a ten out of ten. I am the single user of Microsoft Azure Machine Learning Studio.
How are customer service and support?
We haven't had any experience with the tool's support because we didn't use it. We are mature developers and don't need it at this time. We don't have any complex business needs.
How was the initial setup?
The tool's deployment time depends on the resource you will deploy. Some resources are deployed within minutes, while others may take more than 15-20 minutes. I have deployed mostly web applications, REST APIs, and databases.
What was our ROI?
We're trying to provide robust solutions to our customers, which previously involved multiple steps. Now, we're going to provide it in one step. That is our benefit because the customer will get a final solution, not a solution in steps. We will formalize and streamline them to align with our new solutions.
What's my experience with pricing, setup cost, and licensing?
We pay only the Azure costs for what we use, which involves some subscription costs. But essentially, you pay for what you use. There are no extra costs in addition to the standard licensing fees.
What other advice do I have?
We are trying to find some commercial value. I have learned how to use it, and we will integrate it into the project. That's our next goal.
I rate Microsoft Azure Machine Learning Studio a ten out of ten. If you want to use it, get the certifications, and then work on some projects to gain more experience.
Disclosure: I am a real user, and this review is based on my own experience and opinions.
Owner at Channing Stowell Associates
Has the ability to do templating and transfer it so that we can do multiple types of models and data mining
Pros and Cons
- "The ability to do the templating and be able to transfer it so that I can easily do multiple types of models and data mining is a valuable aspect of this solution. You only have to set up the flows, the templates, and the data once and then you can make modifications and test different segmentations throughout."
- "In terms of improvement, I'd like to have more ability to construct and understand the detailed impact of the variables on the model. Their algorithms are very powerful and they explain overall the net contribution of each of the variables to the solution. In terms of being able to say to people "If you did this, you'll get this much more improvement" it wasn't great."
What is our primary use case?
Developing and operationally implementing a powerful lead scoring model for a major Multiufamily developer and operator of apartment properties throughout major western states. The work included 3 years of data across over 60 properties with more than 500,000 leads and 3 million transactions.
How has it helped my organization?
Increased sales force productivity by permitting them to prioritize activity during peak leasing periods on those leads most likely to close
What is most valuable?
The ability to do the templating and be able to transfer it so that I can easily do multiple types of models and data mining is a valuable aspect of this solution. You only have to set up the flows, the templates, and the data once and then you can make modifications and test different segmentations throughout.
We were working across a number of internal departments as well as some outside departments and this solution made it extremely easy to communicate across functional area because it was all in flow chart and data form so that if somebody had an issue, like changing the data set or something like that, they could point right to it and we could get that handled and incorporated into the model. It's extremely efficient on the computer. We had to do a number of resets on the data in the model and to be able to turn things around and validate the model and the new set in two hours, was just incredible for me.
It was very robust. The ability to move the objects around so easily and then communicate is really its power. Then to be able to show it to the sales and senior management, in terms of what was employed and made it very easy to get my job done.
What needs improvement?
In terms of improvement, I'd like to have more ability to understand the detailed impact of the variables on the model and their interactions. Their algorithms are very powerful and they explain overall the net contribution of each of the variables to the solution. In terms of being able to say to people "If you did this, you'll get this much more improvement" Azure (at least my understanding of it) doesn't provide readily accessible tools to assess from a management perspective the impact of their changing a sinimized, the better.gle value - for instance in closing a lead, decreasing response time by 10%.
I recognize that the multivariate algorithms used from decision trees to neural nets do not readily provide the coefficients for each variable ala the older regression modeling approaches. My experience over my 50 years of developing and implementing predictive models has been that more than half the value of modeling lies in improving management's understanding of the process being modeled, often leading to major organization and operational structure changes. More ability to understand the variables impacting the end result being optimized would be very useful.
For how long have I used the solution?
I have worked extensively with this solution for the last three years.
What do I think about the stability of the solution?
I haven't had any problems with stability.
What do I think about the scalability of the solution?
I didn't have any issues with the scale. we rapidly went from test to full implementation across all datasets.
How are customer service and technical support?
I never had to use technical support.
Which solution did I use previously and why did I switch?
I have used SPSS modeler (part of WATSON really) but because client was a Microsoft shop, I switched to Azure.
How was the initial setup?
I found the setup to be very easy. I've been doing this type of work for 50 years so the modern terminology isn't always the same as what I grew up with. It took me a while to understand that, but the setups were very easy. As with anything, the hardest part is always getting the data together, but the outside consultants had built up a very, very good data warehouse. The ability to manipulate the data and create variables was very nice.
THIS IS THE ONLY MODELING APPROACH THAT EVER WORKED THE VERY TIME I RAN IT!!
What's my experience with pricing, setup cost, and licensing?
Because client isa Microsoft shop, everything was Microsoft in terms of having solutions like Power BI and stuff like that. Azure is very useful and very inexpensive.
What other advice do I have?
The major advice I give is that clients must get the user,somebody who understands the business issues, to be deeply involved with it and the data transformation. Most people don't. And that's true for data science applications. We don't just follow the data in a big pile and remodel, we advance the process that we're modeling. Consider what transformations of the data you need to make it workable and usable.
Remember, over half the initial value of modeling is the strategic understanding provided re the importance of different variables to the model and hence the organizaion's performance. Very often the modeling identifies opportunities for changing structures, decision rules, etc. even prior to the model's actual implementation technically.
I would rate it a nine out of ten.
Which deployment model are you using for this solution?
Private Cloud
If public cloud, private cloud, or hybrid cloud, which cloud provider do you use?
Microsoft Azure
Disclosure: I am a real user, and this review is based on my own experience and opinions.
Associate Director Of Technology at a tech vendor with 10,001+ employees
Has a drag and drop feature and easier learning curve, but the number of algorithms available could still be improved
Pros and Cons
- "In terms of what I found most valuable in Microsoft Azure Machine Learning Studio, I especially love the designer because you can just drag and drop items there and apply the logic that's already available with the designer. I love that I can use the libraries in Microsoft Azure Machine Learning Studio, so I don't have to search for the algorithms and all the relevant libraries because I can see them directly on the designer just by dragging and dropping. Though there's a bit of work during data cleansing, that's normal and can't be avoided. At least it's easy to find the relevant algorithm, apply that algorithm to the data, then get the desired output through Microsoft Azure Machine Learning Studio. I also like the API feature of the solution which is readily available for me to expose the output to any consuming application, so that takes out a lot of headache. Otherwise, I have to have a developer who knows the API, and I have to have an API app, so all that is completely taken care of by the Microsoft Azure Machine Learning Studio designer. With the solution, I can concentrate on how to improve the data quality to get quality recommendations, so this lets me concentrate on my job rather than focusing on the regular development of APIs or the pipelines, in particular, the data pipelines pulling the data from other sources. All the data is taken care of and you can also concentrate on other required auxiliary activities rather than just concentrating on machine learning."
- "As for the areas for improvement in Microsoft Azure Machine Learning Studio, I've provided feedback to Microsoft. My company is a Gold Partner of Microsoft, so I provided my feedback in another forum. Right now, it is the number of algorithms available in the designer that has to be improved, though I'm sure Microsoft does it regularly. When you take a use case approach, Microsoft has done that in a lot of places, but not on the Microsoft Azure Machine Learning Studio designer. When I say use case basis, I meant recommending a product or recommending similar products, so if Microsoft can list out use cases and give me a template, it will save me a lot of time and a lot of work because I don't have to scratch my head on which algorithm is better, and I can go with what's recommended by Microsoft. I'm sure that isn't a big task for the Microsoft team who must have seen thousands of use cases already, so out of that experience if the team can come up with a standard template, I'm sure it'll help a lot of organizations cut down on the development time, as well as going with the best industry-standard algorithms rather than experimenting with mine. What I'd like to see in the next version of Microsoft Azure Machine Learning Studio, apart from the use case template, is the improvement of the availability of libraries. Microsoft should also upgrade the Python versions because the old version of Python is still supported and it takes time for Microsoft to upgrade the support for Python. The pace of upgrading Python versions of Microsoft Azure Machine Learning Studio and making those libraries available should be sped up or increased."
What is most valuable?
In terms of what I found most valuable in Microsoft Azure Machine Learning Studio, I especially love the designer because you can just drag and drop items there and apply the logic that's already available with the designer. I love that I can use the libraries in Microsoft Azure Machine Learning Studio, so I don't have to search for the algorithms and all the relevant libraries because I can see them directly on the designer just by dragging and dropping. Though there's a bit of work during data cleansing, that's normal and can't be avoided. At least it's easy to find the relevant algorithm, apply that algorithm to the data, then get the desired output through Microsoft Azure Machine Learning Studio.
I also like the API feature of the solution which is readily available for me to expose the output to any consuming application, so that takes out a lot of headache. Otherwise, I have to have a developer who knows the API, and I have to have an API app, so all that is completely taken care of by the Microsoft Azure Machine Learning Studio designer. With the solution, I can concentrate on how to improve the data quality to get quality recommendations, so this lets me concentrate on my job rather than focusing on the regular development of APIs or the pipelines, in particular, the data pipelines pulling the data from other sources. All the data is taken care of and you can also concentrate on other required auxiliary activities rather than just concentrating on machine learning.
What needs improvement?
As for the areas for improvement in Microsoft Azure Machine Learning Studio, I've provided feedback to Microsoft. My company is a Gold Partner of Microsoft, so I provided my feedback in another forum. Right now, it is the number of algorithms available in the designer that has to be improved, though I'm sure Microsoft does it regularly.
When you take a use case approach, Microsoft has done that in a lot of places, but not on the Microsoft Azure Machine Learning Studio designer. When I say use case basis, I meant recommending a product or recommending similar products, so if Microsoft can list out use cases and give me a template, it will save me a lot of time and a lot of work because I don't have to scratch my head on which algorithm is better, and I can go with what's recommended by Microsoft.
I'm sure that isn't a big task for the Microsoft team who must have seen thousands of use cases already, so out of that experience if the team can come up with a standard template, I'm sure it'll help a lot of organizations cut down on the development time, as well as going with the best industry-standard algorithms rather than experimenting with mine.
What I'd like to see in the next version of Microsoft Azure Machine Learning Studio, apart from the use case template, is the improvement of the availability of libraries. Microsoft should also upgrade the Python versions because the old version of Python is still supported and it takes time for Microsoft to upgrade the support for Python. The pace of upgrading Python versions of Microsoft Azure Machine Learning Studio and making those libraries available should be sped up or increased.
For how long have I used the solution?
I've been working with Microsoft Azure Machine Learning Studio for nearly two years now.
What do I think about the stability of the solution?
Microsoft Azure Machine Learning Studio is a stable solution. My company is already using it in production. At least customers use the recommendations from Microsoft Azure Machine Learning Studio in production, so the solution is quite stable, at least in cases developed by my company.
What do I think about the scalability of the solution?
Microsoft Azure Machine Learning Studio is a solution that's easy to scale. It's pretty easy because it is hosted on Kubernetes, and there is an option in the portal where I can simply move my plan from standard to enterprise. The solution also has an automatic scaling option available because it is on Kubernetes, so it can scale automatically. I'm seeing that it's quite scalable. This has nothing to do with availability because it just runs in the background, and it is not customer-facing, but the output is customer-facing, so availability is a different case, but in terms of scalability, Microsoft Azure Machine Learning Studio is scalable.
How are customer service and support?
The technical support team for Microsoft Azure Machine Learning Studio was pretty good, though I had to tailor the answers to my requirement, but would rate support a four out of five. Most of the questions my company had, more or less, the support team already experienced, so the team had answers readily available which means there wasn't a need to do a lot of R&D, so getting answers from technical support didn't take a lot of time.
How was the initial setup?
In terms of setting up Microsoft Azure Machine Learning Studio, initially, when my company started, the documentation wasn't so good, but now it has improved. Provisioning the solution only takes a few clicks, so it's no big deal, but setting up the pipelines because no enterprise will have a single environment, you'll have to create multiple pre-production and end production environments, so moving my latest changes to the next environment was a bit of a challenge.
Many terminologies are now in the market such as DevSecOps, and MLOps, so that MLOps documentation was available initially, but it wasn't very explanatory, but now, there's a lot of improvement in the MLOps documentation and that will help me move and propagate my changes from one environment to another.
Microsoft has made improvements into the tutorials, especially on MLOps. Finding MLOps experts in the market was also very tough initially, so my company was trying to learn on the job and do it, so it took some thinking and time, but it's still good because you can learn on the job and do it, but you won't always have the luxury of time to learn it.
What's my experience with pricing, setup cost, and licensing?
In terms of pricing, for any cloud solution, you should know the tricks of the trade and how to use it, otherwise, you'll end up paying a lot of money irrespective of the cloud provider, so at least for Microsoft Azure Machine Learning Studio pricing versus AWS, I would rate it three out of five, with one being the most expensive, and five being the cheapest. It could be cheaper, but you also have to be careful when choosing the plans, for example, consider the architecture and a lot of other factors before choosing your plan, if you don't want to end up paying more. If your cloud provider has an optimizer that seems to be available in every provider, that would keep alerting you in terms of resources not being used as much, then that would help you with budgeting.
Which other solutions did I evaluate?
We evaluated quite a lot of options. We compared Microsoft Azure Machine Learning Studio against Google Cloud and AWS solutions, and there were several others available in the market. I'm trying to recollect the names which we compared the solution with. We did the benchmarking, but we went with Microsoft Azure Machine Learning Studio because our clients and their data were on Azure, though that doesn't necessarily make you go with the solution. After all, you can pull the data from any other cloud as well. For our use case, however, we found many of the things were readily available and the learning curve for Microsoft Azure Machine Learning Studio compared to others was better and easier. We didn't have to search for experts in the market to hire them because we could have our in-house team learn and deliver the solution on the job.
What other advice do I have?
Microsoft Azure Machine Learning Studio is a cloud-native solution. It's completely cloud-based.
My company has eight users of Microsoft Azure Machine Learning Studio.
My rating for Microsoft Azure Machine Learning Studio is seven out of ten.
Which deployment model are you using for this solution?
Public Cloud
Disclosure: My company has a business relationship with this vendor other than being a customer: Partner
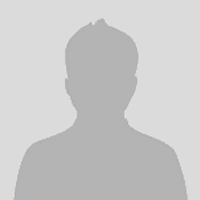
Buyer's Guide
Download our free Microsoft Azure Machine Learning Studio Report and get advice and tips from experienced pros
sharing their opinions.
Updated: March 2025
Popular Comparisons
Amazon SageMaker
IBM SPSS Statistics
Altair RapidMiner
IBM Watson Studio
IBM SPSS Modeler
Domino Data Science Platform
Cloudera Data Science Workbench
SAS Enterprise Miner
Google Cloud Datalab
Buyer's Guide
Download our free Microsoft Azure Machine Learning Studio Report and get advice and tips from experienced pros
sharing their opinions.
Quick Links
Learn More: Questions:
- Which do you prefer - Databricks or Azure Machine Learning Studio?
- What are the biggest differences between Microsoft Azure Machine Learning Studio and TensorFlow?
- What are the pros and cons of Amazon SageMaker vs Microsoft Azure Machine Learning Studio?
- Which are the best end-to-end data science platforms?
- What enterprise data analytics platform has the most powerful data visualization capabilities?
- What Data Science Platform is best suited to a large-scale enterprise?
- When evaluating Data Science Platforms, what aspect do you think is the most important to look for?
- How can ML platforms be used to improve business processes?
- Why is Data Science Platforms important for companies?